Featured
Winning with Data: What’s really important? By Shikha Tandon
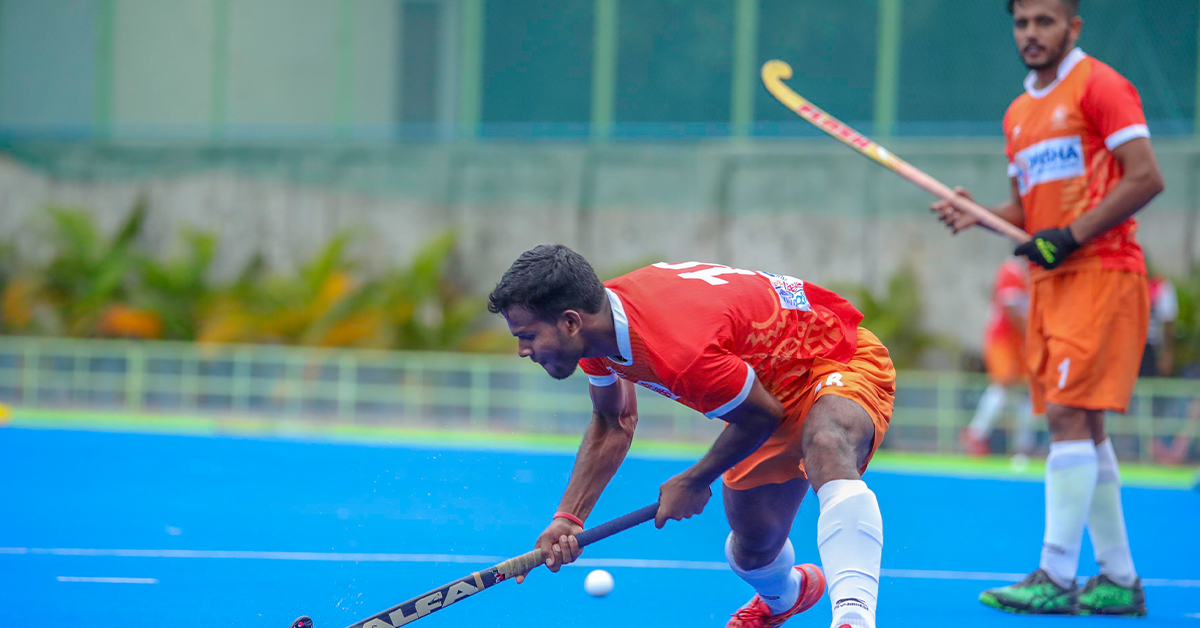
In the previous two articles on optimisation in training and exercise analytics, we discussed the role of technology in our ability to collect data. We live in a world where we have access to constant streams of data from myriad sources; the challenge is translating this data tsunami into actionable insights. However, not all data is relevant or important, and it is vital to be mindful when deciding the key parameters to measure. It’s all about efficiency!
Is it really good to include everything?
There is a common misconception that just by collecting more data you will get a better analysis. The key to generating meaningful insights lies in leveraging specific domain knowledge to identify the relevant metrics, capturing these metrics, and interpreting them. When we consider performance and optimisation tools and products, it is popular to push buzzwords like “Artificial Intelligence (AI)” or “Machine Learning (ML) driven/powered solution/platform”. However, without proper domain knowledge just “throwing AI at the problem” will not result in an optimal analysis. AI can only take into account the inputs it is provided and therefore the output is only as good as the information available to the model. For example, in the case of athletic training, having access to 150 variables of GPS-load but missing the entire areas of sleep, stress, or nutrition will result in sub-optimal recommendations.
Even more important, what outcome is the model being optimised for? In some sports it is pretty easy to decide, like swimming or running, where the time is the final outcome, but how would the model rate performance in team sports? Goals scored would be the easiest to quantify for the team, but clearly not sufficient for an individual player’s optimisation analysis.
Lastly, are all aspects that affect performance included in the model? External factors like weather, time difference, type of competition, etc. could influence performance. A coach could, from empirical knowledge, identify such factors, but the AI may not automatically know to include it.
Contrary to many data and statistical situations, the total amount of data is not the biggest problem in exercise analytics, instead, the crucial factor is availability of accurate relevant data about each specific individual. Current sport science models usually compare group averages, and state if one group/situation/intervention was different from another, but since the objective is to optimise each individual’s performance, having millions of data points for a set of people in different situations, doesn’t help. Instead, having years of data about a single person will help build the best precision algorithms for that individual’s profile.
It is worth noting that there is a balance and a trade off between high accuracy in standardised lab testing and the high frequency option with less accurate ambient measurements. For scientific rigor, the obvious choice is to evaluate, for example a 12-week training program by laboratory tests before and after. Athletes often follow a similar routine with extensive testing a few times a year. Unfortunately, such a setup lacks the ability to guide small adjustments to the training plan. New technologies that enable ambient testing give outcome data from every training session. This is a more beneficial approach for providing exercise analytics; the loss in accuracy is made up by the data frequency.
In addition to technological and computational advances, genetics has a clear role in exercise analytics and is usually considered to account for ~25-50 % of physiological traits. Having said that, most available direct-to-consumer genetic tests provide information about ~10 (up to ~50) genetic variants, which is a very small fraction of the >21,000 genes, and 3 billion base pairs that each of us possesses. This highlights another aspect to consider in exercise analytics, namely the relative contribution of each variable. Commercially available genetic tests are an incomplete indicator of individual performance traits. For coaches and athletes trying to achieve real results, it is much more valuable to measure the outcome (performance) rather than the blueprint (genes). The same holds true for currently available tests for individualised nutrition, microbiome, and other omics.
From data to daily decisions
So how do athletes and coaches actually use all this relevant data we describe above? A whole genre of data-driven decision aid has surfaced over the last couple of years, primarily with a focus on analytics for injury prevention. The long term focus for data-driven decision aids should be to optimise training for each individual. Every elite athlete will have to balance on the edge of too much training while avoiding injuries and illnesses, which is challenging. The quest is to predict when to go even harder and when to focus on recovery. Even more important, to be able to tease out how much of each type of training that specific athlete can tolerate, what sessions give him or her the biggest improvements, and what variables are the most informative for that athlete.
To conclude, in this new era, athletes and coaches should not settle for thoughts and guesses, or inferior or incomplete analytics. Instead they must recognise that, with the right tools and knowledge, exercise analytics can truly individualise and optimise training programs.
This is the third article in the series, titled ‘Winning with data’, on sports science and analytics. The first article discussed optimisation in training, while the second article highlighted the differences between sports analytics and exercise analytics. The authors are founding members of SVEXA, an exercise intelligence company, based in Silicon Valley in the United States, and can be contacted at [email protected]
By Shikha Tandon, Darren Montgomery, Filip Larsen, Daryl Waggott, Euan Ashley & C. Mikael Mattsson.