Featured
Winning with Data: Athlete Profiling | By Shikha Tandon
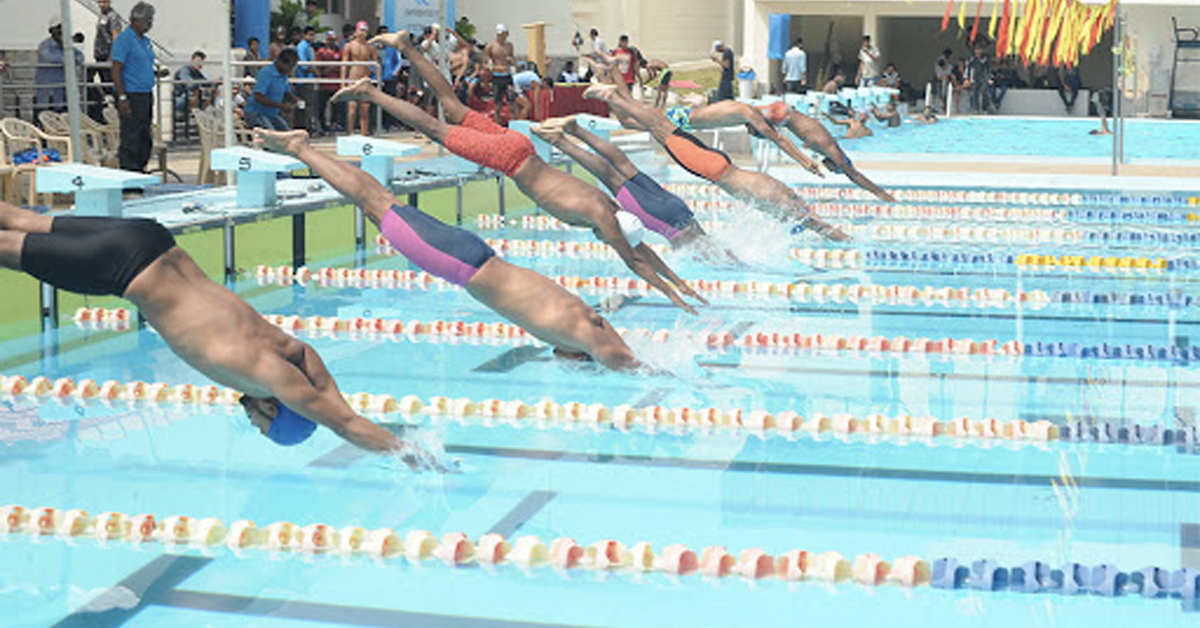
At a time when advances in technology have given us the capability to collect millions of data points on athletes, it is even more crucial to collect and analyse data that is both meaningful and relevant. In most cases, the quantitative scientific method compares groups or situations, like training programs, to each other. The resulting analysis is therefore describing the best option for the majority of people. In reality though, some individuals will have a different or even opposite reaction. A currently often-used example is heart rate variability (HRV), which is the variation in the time interval between heartbeats. A normal healthy heart does not have an even pace, but instead has a constant variation. While a higher variability is preferred, decreases in HRV can help detect an imminent infection (like a cold or COVID-19) or identify excessive training loads. However, data shows that over 20% of individuals have increased HRV or no changes to HRV in these situations. A key aspect of athlete profiling is to ensure that these reactions are consistent, so the individual has the same response every time.
Athlete profiling is a representation of the athlete's individual and stable responses to training and performance. Since there are now thousands of potentially relevant correlations in the data sets, modern athlete profiling is a method to better understand each individual and how the various aspects of their physiology and performance uniquely come together. Similar to precision medicine and precision health, what works for one athlete may not work for another. In order to gain actionable insights, it is vital to highlight the variables that provide the strongest signals for that particular person. This information can be condensed into a format like Svexa’s Player/Athlete Passport®, displaying a selection of the athlete’s most important metrics. One example of a powerful metric that might not be obvious is the relationship between sleep and training load. For some athletes, their sleep is not affected by their training load, but other athletes will get disturbed sleep after really hard training sessions. So instead of meeting the increased need for recovery they get an additional stress, and therefore show increased vulnerability to injuries and overtraining during periods of hard training.
When building athlete profiles, there are a few considerations that may determine the depth of the profile, like budget, athletic level, availability of data, knowledge of the coach, etc. In an ideal world, everything that affects training and performance is included, but don’t be overwhelmed by that unattainable utopia. There is value in using even one marker, and the value and accuracy will increase with every added measurement. All types of stressors should be included, for example academic or work stress, travel, changes in environment (altitude, temperature, city, rural), since the responses to each of these are specific to the individual. For example, for endurance athletes the profile might include the number of days it takes from leaving high altitude training to peak performance at sea level.
As an example of how Svexa uses data to drive insight, we performed analysis on elite swimmers prior to their participation in the 2019 World Championships. We incorporated two years of training, test, and race data to create individualised training optimisation algorithms. The coach had worked with these athletes for several years and had empirical knowledge that showed high diversity of training profiles within the group. Since we had access to training logs, if our athletic profiling was correct, we should be able to predict the upcoming race results. We made the predictions, down to the one-hundredth of a second, the days before the championship, and they turned out to be not far from the actual time clocked by the swimmers. The average error was within +/-0.3%. With this level of accuracy, athlete profiling data can be used to plan training regimens, simulate thousands of different adjustments for each athlete and pick the program that has the highest probability of success, enabling the coach to optimise each individual’s training. This is just one example of what's possible when the correct data sets and domain knowledge come together.
Athlete profiling for recreational and younger athletes
As of now, this type of profiling is mainly privy to elite athletes, and as demonstrated in the above example, exercise intelligence is a powerful tool. But is athlete profiling beneficial to recreational and younger athletes as well? The short answer is YES. Technology has a lot to offer to improve performance for all types of athletes. However, there are certain considerations to be made for younger athletes and recreational athletes.
Typically, junior teams have smaller budgets than professional teams. This will influence their ability to collect data using advanced technology and equipment. However, there are various metrics that do not require expensive equipment for their collection but, when analysed efficiently, can provide intelligence to athletes of every level. The most prominent example is daily subjective ratings including sleep, stress, nutrition, menstruation etc. Another factor is the domain expertise of the coach, and their ability to leverage the captured data and turn it into meaningful insights. While there are many Athlete Management Systems (AMS) that may offer data management services, the real value lies in the ability to derive meaningful insights, hence creating a stronger athlete profile, irrespective of the athlete level.
For younger, age-group athletes, data analytics is already used in technique analysis. As they get older, exercise intelligence can also be used to avoid burnout and fatigue, as well as injury risk assessment and overtraining. At the elite level, these profiles pave the way for more complex analytics to include optimisation and recovery. The type of metrics, be it physiological, technique, or readiness, that have the most impact on athletic performance varies by sport and activity.
A question that gets asked frequently is the role of genetics in determining the above factors for each individual, irrespective of sport. Such questions are commonly asked in the field of athlete talent identification seeking to identify a predisposition to ‘performance’ in a specific sport vs another. In short, yes, the genetic profile will dictate a substantial part of these responses, however, as of today there is absolutely no genetic test that can be used for talent identification. It is important to remember that genetics is the blueprint whereas the physiology is the built structure. If the interest is sprinting ability, the most information is in a sprint test - not a genetic test.
To conclude, there are scientifically sound ways to create and use athlete profiling to predict performance or adjust training programs, including creating risk assessments of injury and illness. Individual models are crucial for all predictions concerning athletes at all levels.
By Shikha Tandon, Darren Montgomery, Filip Larsen, Daryl Waggott, Euan Ashley & C. Mikael Mattsson. This is the penultimate article in the series, titled ‘Winning with data’, on sports science and analytics. The first article discussed optimisation in training, the second article highlighted the differences between sports analytics and exercise analytics, and the third article offered insight into what data is important. The authors are founding members of SVEXA, an exercise intelligence company, based in Silicon Valley in the United States, and can be contacted at [email protected]